Gillespie algorithm
In probability theory, the Gillespie algorithm (or occasionally the Doob-Gillespie algorithm) generates a statistically correct trajectory (possible solution) of a stochastic equation system for which the reaction rates are known. It was created by Joseph L. Doob and others (circa 1945), presented by Dan Gillespie in 1976, and popularized in 1977 in a paper where he uses it to simulate chemical or biochemical systems of reactions efficiently and accurately using limited computational power (see stochastic simulation). As computers have become faster, the algorithm has been used to simulate increasingly complex systems. The algorithm is particularly useful for simulating reactions within cells, where the number of reagents is low and keeping track of the position and behaviour of individual molecules is computationally feasible. Mathematically, it is a variant of a dynamic Monte Carlo method and similar to the kinetic Monte Carlo methods. It is used heavily in computational systems biology.
History
The process that led to the algorithm recognizes several important steps. In 1931, Andrei Kolmogorov introduced the differential equations corresponding to the time-evolution of stochastic processes that proceed by jumps, today known as Kolmogorov equations (Markov jump process) (a simplified version is known as master equation in the natural sciences). It was William Feller, in 1940, who found the conditions under which the Kolmogorov equations admitted (proper) probabilities as solutions. In his Theorem I (1940 work) he establishes that the time-to-the-next-jump was exponentially distributed and the probability of the next event is proportional to the rate. As such, he established the relation of Kolmogorov's equations with stochastic processes. Later, Doob (1942, 1945) extended Feller's solutions beyond the case of pure-jump processes. The method was implemented in computers by David George Kendall (1950) using the Manchester Mark 1 computer and later used by Maurice S. Bartlett (1953) in his studies of epidemics outbreaks. Gillespie (1977) obtains the algorithm in a different manner by making use of a physical argument.
Idea behind the algorithm
Traditional continuous and deterministic biochemical rate equations do not accurately predict cellular reactions since they rely on bulk reactions that require the interactions of millions of molecules. They are typically modeled as a set of coupled ordinary differential equations. In contrast, the Gillespie algorithm allows a discrete and stochastic simulation of a system with few reactants because every reaction is explicitly simulated. A trajectory corresponding to a single Gillespie simulation represents an exact sample from the probability mass function that is the solution of the master equation.
The physical basis of the algorithm is the collision of molecules within a reaction vessel. It is assumed that collisions are frequent, but collisions with the proper orientation and energy are infrequent. Therefore, all reactions within the Gillespie framework must involve at most two molecules. Reactions involving three molecules are assumed to be extremely rare and are modeled as a sequence of binary reactions. It is also assumed that the reaction environment is well mixed.
Algorithm
A recent review (Gillespie, 2007) outlines three different, but equivalent formulations; the direct, first-reaction, and first-family methods, whereby the former two are special cases of the latter. The formulation of the direct and first-reaction methods is centered on performing the usual Monte-Carlo inversion steps on the so-called "fundamental premise of stochastic chemical kinetics", which mathematically is the function
- ,
where each of the terms are propensity functions of an elementary reaction, whose argument is , the vector of species counts. The parameter is the time to the next reaction (or sojourn time), and is of course the current time. To paraphrase Gillespie, this expression is read as "the probability, given , that the system's next reaction will occur in the infinitesimal time interval , and will be of stoichiometry corresponding to the th reaction". This formulation provides a window to the direct and first-reaction methods by implying is an exponentially-distributed random variable, and is "a statistically independent integer random variable with point probabilities ".
Thus, the Monte-Carlo generating method is simply to draw two pseudorandom numbers, and on , and compute
- ,
and
- the smallest integer satisfying .
Utilizing this generating method for the sojourn time and next reaction, the direct method algorithm is stated by Gillespie as
1. Initialize the time and the system's state 2. With the system in state at time , evaluate all the and their sum 3. Effect the next reaction by replacing and 4. Record as desired. Return to step 1, or else end the simulation.
This family of algorithms is computationally expensive and thus many modifications and adaptations exist, including the next reaction method (Gibson & Bruck), tau-leaping, as well as hybrid techniques where abundant reactants are modeled with deterministic behavior. Adapted techniques generally compromise the exactitude of the theory behind the algorithm as it connects to the Master equation, but offer reasonable realizations for greatly improved timescales. The computational cost of exact versions of the algorithm is determined by the coupling class of the reaction network. In weakly coupled networks, the number of reactions that is influenced by any other reaction is bounded by a small constant. In strongly coupled networks, a single reaction firing can in principle affect all other reactions. An exact version of the algorithm with constant-time scaling for weakly coupled networks has been developed, enabling efficient simulation of systems with very large numbers of reaction channels (Slepoy Thompson Plimpton 2008). The generalized Gillespie algorithm that accounts for the non-Markovian properties of random biochemical events with delay has been developed by Bratsun et al. 2005 and independently Barrio et al. 2006, as well as (Cai 2007). See the articles cited below for details.
Partial-propensity formulations, as developed independently by both Ramaswamy et al. (2009, 2010) and Indurkhya and Beal (2010), are available to construct a family of exact versions of the algorithm whose computational cost is proportional to the number of chemical species in the network, rather than the (larger) number of reactions. These formulations can reduce the computational cost to constant-time scaling for weakly coupled networks and to scale at most linearly with the number of species for strongly coupled networks. A partial-propensity variant of the generalized Gillespie algorithm for reactions with delays has also been proposed (Ramaswamy Sbalzarini 2011). The use of partial-propensity methods is limited to elementary chemical reactions, i.e., reactions with at most two different reactants. Every non-elementary chemical reaction can be equivalently decomposed into a set of elementary ones, at the expense of a linear (in the order of the reaction) increase in network size.
Consider the following object-oriented implementation of the direct- and first-reaction methods, which are contained in a Python 3 class:
from math import log
class SSA:
"""Container for SSAs"""
def __init__(self, model, seed=1234):
"""Initialize container with model and pseudorandom number generator"""
self.model = model
self.random = Mersenne(seed=seed)
def direct(self):
"""Indefinite generator of direct-method trajectories"""
while True:
while not self.model.exit():
# evaluate weights and partition
weights = [
(rxn, sto, pro(self.model))
for (rxn, sto, pro) in self.model.reactions
]
partition = sum(w[-1] for w in weights)
# evaluate sojourn time (MC step 1)
sojourn = log(1.0 / self.random.floating()) / partition
self.model["time"].append(self.model["time"][-1] + sojourn)
# evaluate the reaction (MC step 2)
partition = partition * self.random.floating()
while partition >= 0.0:
rxn, sto, pro = weights.pop(0)
partition -= pro
for species, delta in sto.items():
self.model[species].append(self.model[species][-1] + delta)
self.model.curate()
yield self.model
self.model.reset()
def first_reaction(self):
"""Indefinite generator of 1st-reaction trajectories"""
while True:
while not self.model.exit():
# evaluate next reaction times
times = [
(
log(
1.0 / self.random.floating()
) / pro(self.model),
sto
)
for (rxn, sto, pro) in self.model.reactions
]
times.sort()
# evaluate reaction time
self.model["time"].append(
self.model["time"][-1] + times[0][0]
)
# evaluate reaction
for species, delta in times[0][1].items():
self.model[species].append(
self.model[species][-1] + delta
)
self.model.curate()
yield self.model
self.model.reset()
As can be seen, like all Monte-Carlo methods, SSA
requires a seed for reproducibility, which is passed to the name Mersenne
, a pseudorandom number generator. The implementation of Mersenne
can be found in the Mersenne Twister article, but another can be used, such as random.random
. The direct
and first_reaction
members are indefinite generators, meaning they will continuously produce trajectories, each trajectory being a complete simulation of the system, in a loop until a signal breaks that loop. To actually implement such a loop and yield some number of trajectories for analysis, this class requires a model be passed to it on instantiation. The purpose of the model class is to avoid commingling the kinetic properties of the specific process being simulated with the Gillespie algorithm's logic. A model should be a dictionary subclass with public members curate
, exit
, and reset
that respectively
- determine which reactions are and are not valid at the end of each iteration of a given trajectory;
- return
True
if there are no more possible reactions; - return the model hashings to their original values (i.e. their initial conditions) at the end of a given trajectory.
Consider the following model implementation, which is used in the SIR example below:
class SSAModel(dict):
"""Container for SSA model"""
def __init__(
self, initial_conditions, propensities, stoichiometry
):
"""
Initialize model with a dictionary of initial conditions (each
"""
super().__init__(**initial_conditions)
self.reactions = list()
self.excluded_reactions = list()
for reaction,propensity in propensities.items():
if propensity(self) == 0.0:
self.excluded_reactions.append(
(
reaction,
stoichiometry[reaction],
propensity
)
)
else:
self.reactions.append(
(
reaction,
stoichiometry[reaction],
propensity
)
)
def exit(self):
"""Return True to break out of trajectory"""
# return True if no more reactions
if len(self.reactions) == 0: return True
# return False if there are more reactions
else: return False
def curate(self):
"""Validate and invalidate model reactions"""
# evaulate possible reactions
reactions = []
while len(self.reactions) > 0:
reaction = self.reactions.pop()
if reaction[2](self) == 0:
self.excluded_reactions.append(reaction)
else:
reactions.append(reaction)
reactions.sort()
self.reactions = reactions
# evaluate impossible reactions
excluded_reactions = []
while len(self.excluded_reactions) > 0:
reaction = self.excluded_reactions.pop()
if reaction[2](self) > 0:
self.reactions.append(reaction)
else:
excluded_reactions.append(reaction)
excluded_reactions.sort()
self.excluded_reactions = excluded_reactions
def reset(self):
"""Clear the trajectory"""
# reset species to initial conditions
for key in self: del self[key][1:]
# reset reactions per initial conditions
self.curate()
Examples
Reversible binding of A and B to form AB dimers
A simple example may help to explain how the Gillespie algorithm works. Consider a system of molecules of two types, A and B. In this system, A and B reversibly bind together to form AB dimers such that two reactions are possible: either A and B react reversibly to form an AB dimer, or an AB dimer dissociates into A and B. The reaction rate constant for a given single A molecule reacting with a given single B molecule is , and the reaction rate for an AB dimer breaking up is .
If at time t there is one molecule of each type then the rate of dimer formation is , while if there are molecules of type A and molecules of type B, the rate of dimer formation is . If there are dimers then the rate of dimer dissociation is .
The total reaction rate, , at time t is then given by
So, we have now described a simple model with two reactions. This definition is independent of the Gillespie algorithm. We will now describe how to apply the Gillespie algorithm to this system.
In the algorithm, we advance forward in time in two steps: calculating the time to the next reaction, and determining which of the possible reactions the next reaction is. Reactions are assumed to be completely random, so if the reaction rate at a time t is , then the time, δt, until the next reaction occurs is a random number drawn from exponential distribution function with mean . Thus, we advance time from t to t + δt.
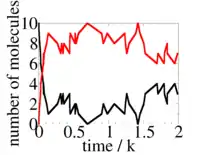
The probability that this reaction is an A molecule binding to a B molecule is simply the fraction of total rate due to this type of reaction, i.e.,
the probability that reaction is
The probability that the next reaction is an AB dimer dissociating is just 1 minus that. So with these two probabilities we either form a dimer by reducing and by one, and increase by one, or we dissociate a dimer and increase and by one and decrease by one.
Now we have both advanced time to t + δt, and performed a single reaction. The Gillespie algorithm just repeats these two steps as many times as needed to simulate the system for however long we want (i.e., for as many reactions). The result of a Gillespie simulation that starts with and at t=0, and where and , is shown at the right. For these parameter values, on average there are 8 dimers and 2 of A and B but due to the small numbers of molecules fluctuations around these values are large. The Gillespie algorithm is often used to study systems where these fluctuations are important.
That was just a simple example, with two reactions. More complex systems with more reactions are handled in the same way. All reaction rates must be calculated at each time step, and one chosen with probability equal to its fractional contribution to the rate. Time is then advanced as in this example.
The SIR epidemic without vital dynamics
The SIR model is a classic biological description of how certain diseases permeate through a fixed-size population. In its simplest form there are members of the population, whereby each member may be in one of three states—susceptible, infected, or recovered—at any instant in time, and each such member transitions irreversibly through these states according to the directed graph below. We can denote the number of susceptible members as , the number of infected members as , and the number of recovered members as . Therefore for any point in time.

Further, a given susceptible member will transition to the infected state by coming into contact with any of the infected members, so infection occurs with rate . A given member of the infected state recovers without dependence on any of the three states, which is specified by rate β. Given this basic scheme, it possible to construct the following non-linear system.
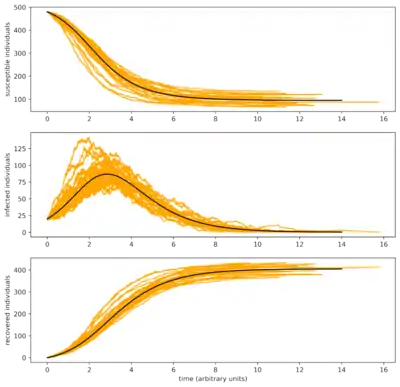
- ,
- ,
- .
This system has no analytical solution. One approach could be to use the Gillespie algorithm, simulate the system many times, and use a regression technique such as least-squares to fit a polynomial over all of the trajectories. As the number of trajectories increases, such polynomial regression will asymptotically behave like the numerical solution (black lines). In addition to estimating the solution of an intractable problem like the SIR epidemic, the stochastic nature of each trajectory allows one to compute statistics other than . When running the script below, sometimes realizations of the SIR epidemic are obtained which differ drastically from the numerical solution. For example, when all persons are cured (by chance) very early, or very late.
The trajectories presented in the above figure were obtained with the following Python implementation of the direct method, along with a model class whose members interact with the direct method machinery to perform general simulations with the theories underlying the Gillespie algorithm. These are introduced above. Additionally, an ODE solver from SciPy is invoked to obtain the numerical solution of the differential equation system, i.e. a representation of .
from matplotlib import pyplot
from numpy import linspace
from scipy.integrate import odeint
# initial species counts and sojourn times
initital_conditions = {
"s": [480],
"i": [20],
"r": [0],
"time": [0.0],
}
# propensity functions
propensities = {
0: lambda d: 2.0 * d["s"][-1] * d["i"][-1] / 500,
1: lambda d: 1.0 * d["i"][-1],
}
# change in species for each propensity
stoichiometry = {
0: {"s": -1, "i": 1, "r": 0},
1: {"s": 0, "i": -1, "r": 1},
}
# instantiate the epidemic SSA model container
epidemic = SSAModel(
initital_conditions,
propensities,
stoichiometry
)
# instantiate the SSA container with model
epidemic_generator = SSA(epidemic)
# make a nice, big figure
pyplot.figure(figsize=(10,10), dpi=500)
# make a subplot for the susceptible, infected and recovered individuals
axes_s = pyplot.subplot(311)
axes_s.set_ylabel("susceptible individuals")
axes_i = pyplot.subplot(312)
axes_i.set_ylabel("infected individuals")
axes_r = pyplot.subplot(313)
axes_r.set_ylabel("recovered individuals")
axes_r.set_xlabel("time (arbitrary units)")
# simulate and plot 30 trajectories
trajectories = 0
for trajectory in epidemic_generator.direct():
axes_s.plot(trajectory["time"], trajectory["s"], color="orange")
axes_i.plot(trajectory["time"], trajectory["i"], color="orange")
axes_r.plot(trajectory["time"], trajectory["r"], color="orange")
trajectories += 1
if trajectories == 30:
break
# numerical solution using an ordinary differential equation solversir
t = linspace(0, 14, num=200)
y0 = (480, 20, 0)
alpha = 2.0
beta = 1.0
def differential_SIR(n_SIR, t, alpha, beta):
dS_dt = -alpha * n_SIR[0] * n_SIR[1] / 500
dI_dt = ((alpha * n_SIR[0] / 500) - beta) * n_SIR[1]
dR_dt = beta * n_SIR[1]
return dS_dt, dI_dt, dR_dt
solution = odeint(differential_SIR, y0, t, args=(alpha, beta))
solution = [[row[i] for row in solution] for i in range(3)]
# plot numerical solution
axes_s.plot(t, solution[0], color="black")
axes_i.plot(t, solution[1], color="black")
axes_r.plot(t, solution[2], color="black")
pyplot.show()
Further reading
- Gillespie, Daniel T. (1977). "Exact Stochastic Simulation of Coupled Chemical Reactions". The Journal of Physical Chemistry. 81 (25): 2340–2361. CiteSeerX 10.1.1.704.7634. doi:10.1021/j100540a008.
- Gillespie, Daniel T. (1976). "A General Method for Numerically Simulating the Stochastic Time Evolution of Coupled Chemical Reactions". Journal of Computational Physics. 22 (4): 403–434. Bibcode:1976JCoPh..22..403G. doi:10.1016/0021-9991(76)90041-3.
- Gibson, Michael A.; Bruck, Jehoshua (2000). "Efficient Exact Stochastic Simulation of Chemical Systems with Many Species and Many Channels" (PDF). Journal of Physical Chemistry A. 104 (9): 1876–1889. Bibcode:2000JPCA..104.1876G. doi:10.1021/jp993732q.
- Doob, Jacob L. (1942). "Topics in the Theory of Markoff Chains". Transactions of the American Mathematical Society. 52 (1): 37–64. doi:10.1090/S0002-9947-1942-0006633-7. JSTOR 1990152.
- Doob, Jacob L. (1945). "Markoff chains – Denumerable case". Transactions of the American Mathematical Society. 58 (3): 455–473. doi:10.2307/1990339. JSTOR 1990339.
- Press, William H.; Teukolsky, Saul A.; Vetterling, William T.; Flannery, Brian P. (2007). "Section 17.7. Stochastic Simulation of Chemical Reaction Networks". Numerical Recipes: The Art of Scientific Computing (3rd ed.). New York, NY: Cambridge University Press. ISBN 978-0-521-88068-8.
- Kolmogorov, Andrey N. (1931). "Über die analytischen Methoden in der Wahrscheinlichkeitsrechnung" [On Analytical Methods in the Theory of Probability]. Mathematische Annalen. 104: 415–458. doi:10.1007/BF01457949. S2CID 119439925.
- Feller, Willy (1940). "On the Integro-Differential Equations of Purely Discontinuous Markoff Processes". Transactions of the American Mathematical Society. 48 (3): 4885–15. doi:10.2307/1990095. JSTOR 1970064.
- Kendall, David G. (1950). "An Artificial Realization of a Simple "Birth-and-Death" Process". Journal of the Royal Statistical Society, Series B. 12 (1): 116–119. JSTOR 2983837.
- Bartlett, Maurice S. (1953). "Stochastic Processes or the Statistics of Change". Journal of the Royal Statistical Society, Series C. 2 (1): 44–64. JSTOR 2985327.
- Rathinam, Muruhan; Petzold, Linda R.; Cao, Yang; Gillespie, Daniel T. (2003). "Stiffness in stochastic chemically reacting systems: The implicit tau-leaping method". Journal of Chemical Physics. 119 (24): 12784–12794. Bibcode:2003JChPh.11912784R. doi:10.1063/1.1627296.
- Sinitsyn, Nikolai A.; Hengartner, Nicolas; Nemenman, Ilya (2009). "Adiabatic coarse-graining and simulations of stochastic biochemical networks" (PDF). Proceedings of the National Academy of Sciences of the United States of America. 106 (20): 10546–10551. Bibcode:2009PNAS..10610546S. doi:10.1073/pnas.0809340106. PMC 2705573. PMID 19525397. Archived from the original (PDF) on 2011-07-14.
- Salis, Howard; Kaznessis, Yiannis N. (2005). "Accurate hybrid stochastic simulation of a system of coupled chemical or biochemical reactions". Journal of Chemical Physics. 122 (5): 054103. Bibcode:2005JChPh.122e4103S. doi:10.1063/1.1835951. PMID 15740306.
- (Slepoy Thompson Plimpton 2008): Slepoy, Alexander; Thompson, Aidan P.; Plimpton, Steven J. (2008). "A constant-time kinetic Monte Carlo algorithm for simulation of large biochemical reaction networks". Journal of Chemical Physics. 128 (20): 205101. Bibcode:2008JChPh.128t5101S. doi:10.1063/1.2919546. PMID 18513044.
- (Bratsun et al. 2005): Bratsun, Dmitri; Volfson, Dmitri; Hasty, Jeff; Tsimring, Lev S. (2005). "Delay-induced stochastic oscillations in gene regulation". Proceedings of the National Academy of Sciences of the United States of America. 102 (41): 14593–8. Bibcode:2005PNAS..10214593B. doi:10.1073/pnas.0503858102. PMC 1253555. PMID 16199522.
- (Barrio et al. 2006): Barrio, Manuel; Burrage, Kevin; Leier, André; Tian, Tianhai (2006). "Oscillatory Regulation of hes1: Discrete Stochastic Delay Modelling and Simulation". PLOS Computational Biology. 2 (9): 1017. Bibcode:2006PLSCB...2..117B. doi:10.1371/journal.pcbi.0020117. PMC 1560403. PMID 16965175.
- (Cai 2007): Cai, Xiaodong (2007). "Exact stochastic simulation of coupled chemical reactions with delays". Journal of Chemical Physics. 126 (12): 124108. Bibcode:2007JChPh.126l4108C. doi:10.1063/1.2710253. PMID 17411109.
- (Barnes Chu 2010): Barnes, David J.; Chu, Dominique (2010). Introduction to Modeling for Biosciences. Springer Verlag.
- (Ramaswamy González-Segredo Sbalzarini 2009): Ramaswamy, Rajesh; González-Segredo, Nélido; Sbalzarini, Ivo F. (2009). "A new class of highly efficient exact stochastic simulation algorithms for chemical reaction networks". Journal of Chemical Physics. 130 (24): 244104. arXiv:0906.1992. Bibcode:2009JChPh.130x4104R. doi:10.1063/1.3154624. PMID 19566139. S2CID 4952205.
- (Ramaswamy Sbalzarini 2010): Ramaswamy, Rajesh; Sbalzarini, Ivo F. (2010). "A partial-propensity variant of the composition-rejection stochastic simulation algorithm for chemical reaction networks" (PDF). Journal of Chemical Physics. 132 (4): 044102. Bibcode:2010JChPh.132d4102R. doi:10.1063/1.3297948. PMID 20113014.
- (Indurkhya Beal 2010): Indurkhya, Sagar; Beal, Jacob S. (2005). Isalan, Mark (ed.). "Reaction Factoring and Bipartite Update Graphs Accelerate the Gillespie Algorithm for Large-Scale Biochemical Systems". PLOS ONE. 5 (1): e8125. Bibcode:2010PLoSO...5.8125I. doi:10.1371/journal.pone.0008125. PMC 2798956. PMID 20066048.
- (Ramaswamy Sbalzarini 2011): Ramaswamy, Rajesh; Sbalzarini, Ivo F. (2011). "A partial-propensity formulation of the stochastic simulation algorithm for chemical reaction networks with delays" (PDF). Journal of Chemical Physics. 134 (1): 014106. Bibcode:2011JChPh.134a4106R. doi:10.1063/1.3521496. PMID 21218996.
- (Yates Klingbeil 2013): Yates, Christian A.; Klingbeil, Guido (2013). "Recycling random numbers in the stochastic simulation algorithm". Annual Review of Physical Chemistry. 58 (9): 094103. Bibcode:2013JChPh.138i4103Y. doi:10.1063/1.4792207. PMID 23485273.
- Gillespie, Daniel T. (2007). "Stochastic Simulation of Chemical Kinetics". The Journal of Physical Chemistry. 58: 35–55. doi:10.1146/annurev.physchem.58.032806.104637.